Multivariate Distributions#
Key concepts for this section:
Correlation / covariance
Contingency Table
Plotting joint/conditional/marginal distributions
Simpson’s paradox, confounder?
Show code cell content
from IPython import get_ipython
if get_ipython() is not None:
get_ipython().run_line_magic('load_ext', 'autoreload')
get_ipython().run_line_magic('autoreload', '2')
import seaborn as sns; sns.set()
import matplotlib.pyplot as plt
import numpy as np
import pandas as pd
from scipy import stats
sns.set(rc={'figure.figsize':(9,4)})
# Fake Data:
n=100
df = pd.DataFrame({
"a":np.random.normal(10, 1, n),
})
df["c"] = df["a"] + np.random.normal(1, 0.5, n)
Correlation / Covariance#
Covariance indicates the level to which two variables vary together.
\[Cov(X,Y) = \frac{1}{n}\sum_{i=1}^n(x_i - E(X))(y_i-E(Y))\]
# Compute covariance manually:
products = [
(row["a"]-df["a"].mean()) * (row["c"]-df["c"].mean())
for i,row in df.iterrows()
]
covariance = np.sum(products)/len(df)
print(covariance)
1.2540311514944218
# Using numpy:
# if bias = False, computes "sample covariance" so denominator is N-1
np.cov(df["a"], df["c"], bias=True)
array([[1.25936321, 1.25403115],
[1.25403115, 1.46689568]])
Pearson correlation coefficient is the covariance divided by product of standard deviations
\[\rho_{X,Y} = \frac{cov(X,Y)}{\sigma_X\sigma_Y}\]
also see: spearman’s rank correlation coefficient
corr = covariance / (np.std(df["a"]) * np.std(df["c"]))
corr
0.9226419733025584
np.corrcoef(df["a"], df["c"])
array([[1. , 0.92264197],
[0.92264197, 1. ]])
Contingency Table#
df_cat = pd.DataFrame({
"colors":np.random.choice(["red","blue","green","yellow","orange"], size=30, replace=True),
"names":np.random.choice(["jason","jorge","lisa","paul"], size=30, replace=True),
"states":np.random.choice(["california","arizona","oregon"], size=30, replace=True),
})
pd.crosstab(
[df_cat["states"],df_cat["names"]],
df_cat["colors"],
margins=True
)
colors | blue | green | orange | red | yellow | All | |
---|---|---|---|---|---|---|---|
states | names | ||||||
arizona | jason | 1 | 0 | 1 | 0 | 1 | 3 |
jorge | 0 | 0 | 0 | 0 | 1 | 1 | |
lisa | 0 | 0 | 0 | 1 | 0 | 1 | |
paul | 3 | 0 | 0 | 1 | 0 | 4 | |
california | jason | 0 | 0 | 1 | 1 | 1 | 3 |
jorge | 1 | 0 | 0 | 1 | 1 | 3 | |
lisa | 0 | 2 | 0 | 1 | 1 | 4 | |
paul | 0 | 0 | 1 | 1 | 1 | 3 | |
oregon | jason | 0 | 1 | 1 | 1 | 1 | 4 |
lisa | 1 | 0 | 1 | 2 | 0 | 4 | |
All | 6 | 3 | 5 | 9 | 7 | 30 |
pd.crosstab(
[df_cat["states"],df_cat["names"]], df_cat["colors"],
margins=True,
normalize=True
)
colors | blue | green | orange | red | yellow | All | |
---|---|---|---|---|---|---|---|
states | names | ||||||
arizona | jason | 0.033333 | 0.000000 | 0.033333 | 0.000000 | 0.033333 | 0.100000 |
jorge | 0.000000 | 0.000000 | 0.000000 | 0.000000 | 0.033333 | 0.033333 | |
lisa | 0.000000 | 0.000000 | 0.000000 | 0.033333 | 0.000000 | 0.033333 | |
paul | 0.100000 | 0.000000 | 0.000000 | 0.033333 | 0.000000 | 0.133333 | |
california | jason | 0.000000 | 0.000000 | 0.033333 | 0.033333 | 0.033333 | 0.100000 |
jorge | 0.033333 | 0.000000 | 0.000000 | 0.033333 | 0.033333 | 0.100000 | |
lisa | 0.000000 | 0.066667 | 0.000000 | 0.033333 | 0.033333 | 0.133333 | |
paul | 0.000000 | 0.000000 | 0.033333 | 0.033333 | 0.033333 | 0.100000 | |
oregon | jason | 0.000000 | 0.033333 | 0.033333 | 0.033333 | 0.033333 | 0.133333 |
lisa | 0.033333 | 0.000000 | 0.033333 | 0.066667 | 0.000000 | 0.133333 | |
All | 0.200000 | 0.100000 | 0.166667 | 0.300000 | 0.233333 | 1.000000 |
Joint / Conditional / Marginal Distributions#
joint distribution
\[f(a,c)\]
sns.jointplot(df["a"],df["c"])
/home/chansoo/projects/statsbook/.venv/lib/python3.8/site-packages/seaborn/_decorators.py:36: FutureWarning: Pass the following variables as keyword args: x, y. From version 0.12, the only valid positional argument will be `data`, and passing other arguments without an explicit keyword will result in an error or misinterpretation.
warnings.warn(
<seaborn.axisgrid.JointGrid at 0x7f3518532640>
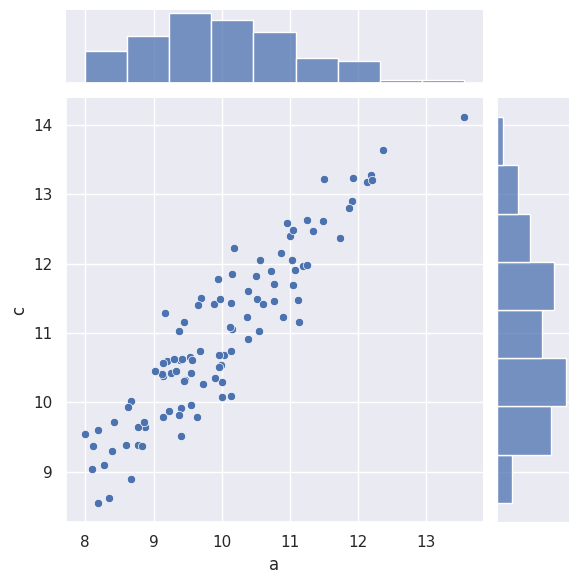
conditional distribution
\[f(a|c>0)\]
sns.kdeplot(df.loc[df["c"]>0,"a"])
<Axes: xlabel='a', ylabel='Density'>
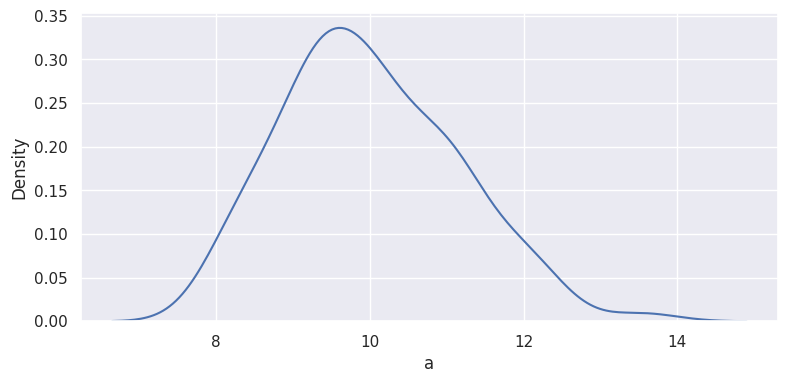
marginal distribution
\[f(a)\]
sns.kdeplot(df["a"])
<Axes: xlabel='a', ylabel='Density'>
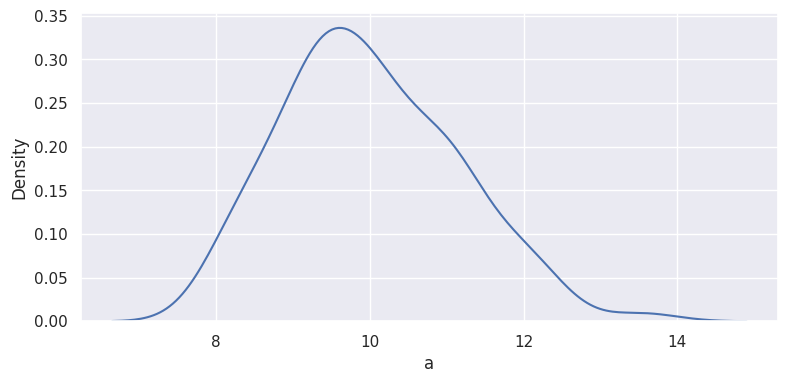
Simpson’s Paradox#
df2 = pd.DataFrame({
"a":np.random.normal(8, 1, n),
})
df2["c"] = df2["a"] + np.random.normal(8, 0.5, n)
df["group"] = 0
df2["group"] = 1
df_simpson = pd.concat([df[["a","c","group"]],df2])
sns.regplot(df_simpson["a"], df_simpson["c"], scatter=False, color="red")
sns.scatterplot(df_simpson["a"], df_simpson["c"], hue=df_simpson["group"])
/home/chansoo/projects/statsbook/.venv/lib/python3.8/site-packages/seaborn/_decorators.py:36: FutureWarning: Pass the following variables as keyword args: x, y. From version 0.12, the only valid positional argument will be `data`, and passing other arguments without an explicit keyword will result in an error or misinterpretation.
warnings.warn(
<Axes: xlabel='a', ylabel='c'>
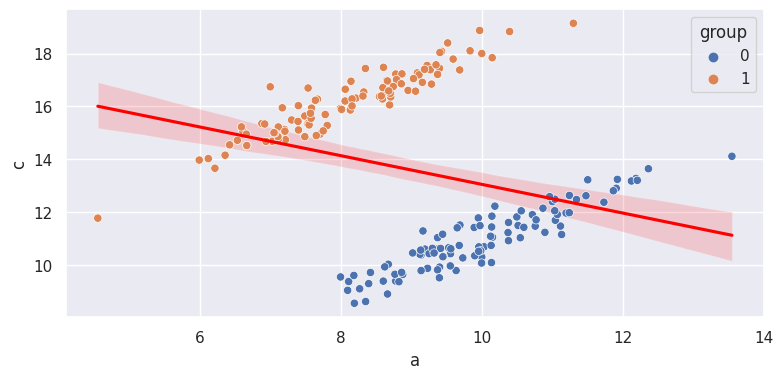