Discriminant Analysis#
Discriminant Analysis is used for classification when your data are continuous variables. The key assumptions are multivariate normality and homoscedasticity.
Linear Discriminant Analysis (LDA) assumes for each class, observations are drawn from \(N(\mu_k, \Sigma)\) for class k and that \(\Sigma\) is constant across all classes
Using Bayes (f(x) ~ N(\mu, \Sigmaa)): \(P(Y=k | X=x) = \frac{\pi_kf_k(x)}{\sum_{i=1}^{K}\pi_i f_i(x)}\)
Taking log of above, Bayes classifier assigns an observation to the class for which the following is maximized: \(x^T\Sigma^{-1}\mu_k-\frac{1}{2}\mu_k^T\Sigma^{-1}\mu_k + log\ \pi_k\)
Quadratic Discriminant Analysis (QDA) relaxes the homoscedasticity assumption, s.t. instead of a constant \(\Sigma\) across all classes, \(\Sigma_k\) is modeled for each class.
from IPython import get_ipython
if get_ipython() is not None:
get_ipython().run_line_magic('load_ext', 'autoreload')
get_ipython().run_line_magic('autoreload', '2')
import numpy as np
import seaborn as sns
import pandas as pd
from sklearn.discriminant_analysis import LinearDiscriminantAnalysis, QuadraticDiscriminantAnalysis
from sklearn.metrics import confusion_matrix, classification_report
import matplotlib.pyplot as plt
from matplotlib import colors
sns.set(rc={'figure.figsize':(11.7,8.27)})
# Generate Fake Data
np.random.seed(2)
df = pd.DataFrame({
"y":np.repeat(["a","b"], repeats=50)
})
means1 = [-10,10]
means2 = [-13,13]
covmat = [
[1,0.3],
[0.3,1]
]
covmat2 = [
[3,5],
[-4,1]
]
df[["x0", "x1"]] = np.concatenate((
np.random.multivariate_normal(means1,covmat,50),
np.random.multivariate_normal(means2,covmat2,50)
), axis=0)
sns.scatterplot(df["x0"], df["x1"], hue=df["y"])
/tmp/ipykernel_92955/1864784404.py:22: RuntimeWarning: covariance is not symmetric positive-semidefinite.
np.random.multivariate_normal(means2,covmat2,50)
/home/chansoo/projects/statsbook/.venv/lib/python3.8/site-packages/seaborn/_decorators.py:36: FutureWarning: Pass the following variables as keyword args: x, y. From version 0.12, the only valid positional argument will be `data`, and passing other arguments without an explicit keyword will result in an error or misinterpretation.
warnings.warn(
<Axes: xlabel='x0', ylabel='x1'>
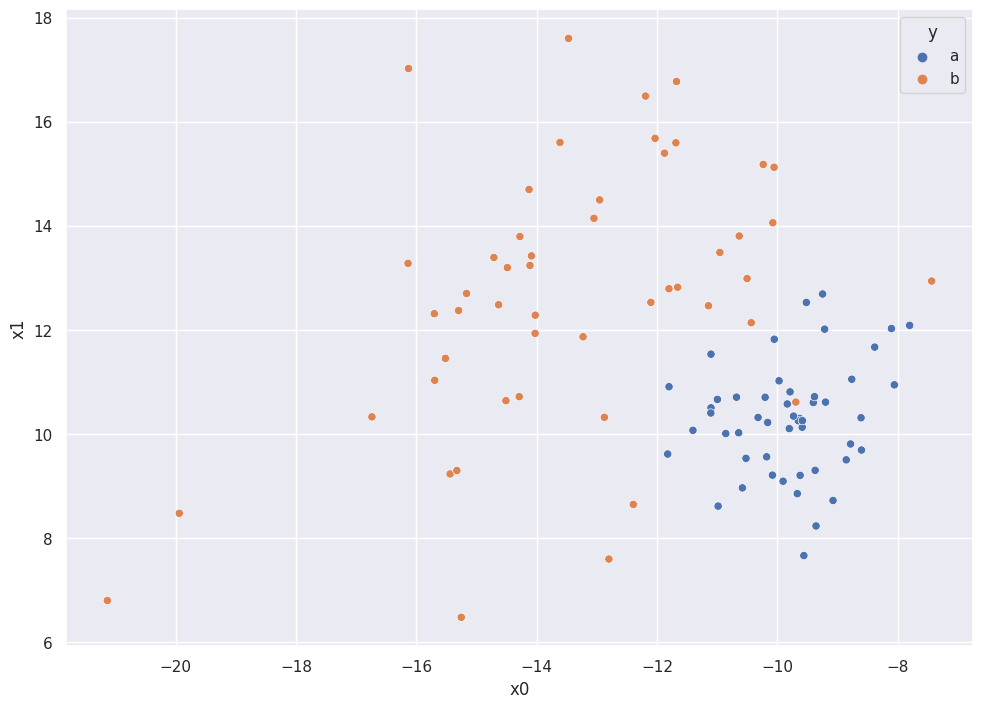
LDA Demonstration#
# Fit LDA Model
lda = LinearDiscriminantAnalysis()
lda.fit(df[["x0","x1"]], df["y"])
w0, w1, b = lda.coef_[0][0], lda.coef_[0][1], lda.intercept_[0]
# Plot Decision Boundary
xx, yy = np.meshgrid(
np.linspace(df["x0"].min(), df["x0"].max(), 200),
np.linspace(df["x1"].min(), df["x1"].max(), 100),
)
Z = lda.predict_proba(np.c_[xx.ravel(), yy.ravel()])
Z = Z[:, 1].reshape(xx.shape)
# g = sns.lineplot(line["x0"], line["x1"])
plt.pcolormesh(
xx, yy, Z, cmap=plt.get_cmap("bwr"), zorder=0, alpha=0.4
)
plt.contour(xx, yy, Z, [0.5], linewidths=2.0, colors="white")
g = sns.scatterplot(df["x0"], df["x1"], hue=df["y"])
/home/chansoo/projects/statsbook/.venv/lib/python3.8/site-packages/sklearn/base.py:439: UserWarning: X does not have valid feature names, but LinearDiscriminantAnalysis was fitted with feature names
warnings.warn(
/home/chansoo/projects/statsbook/.venv/lib/python3.8/site-packages/seaborn/_decorators.py:36: FutureWarning: Pass the following variables as keyword args: x, y. From version 0.12, the only valid positional argument will be `data`, and passing other arguments without an explicit keyword will result in an error or misinterpretation.
warnings.warn(
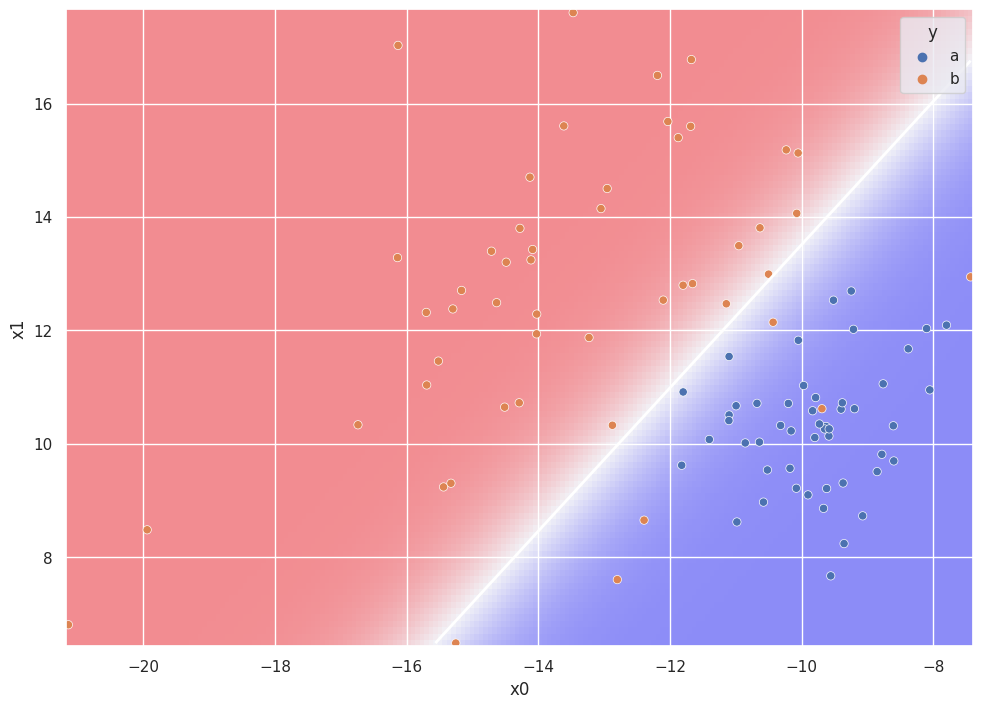
Model Evaluation#
# prediction
y_pred = lda.predict(df[["x0","x1"]])
#error rate
print(np.mean(df["y"]!=y_pred))
print(confusion_matrix(df["y"], y_pred))
# recall of the negative class is "specificity"
print(classification_report(df["y"], y_pred))
0.06
[[50 0]
[ 6 44]]
precision recall f1-score support
a 0.89 1.00 0.94 50
b 1.00 0.88 0.94 50
accuracy 0.94 100
macro avg 0.95 0.94 0.94 100
weighted avg 0.95 0.94 0.94 100
QDA Demonstration#
# Fit LDA Model
qda = QuadraticDiscriminantAnalysis()
qda.fit(df[["x0","x1"]], df["y"])
QuadraticDiscriminantAnalysis()In a Jupyter environment, please rerun this cell to show the HTML representation or trust the notebook.
On GitHub, the HTML representation is unable to render, please try loading this page with nbviewer.org.
QuadraticDiscriminantAnalysis()
labels = qda.predict(df[["x0","x1"]])
# Plot Decision Boundary
xx, yy = np.meshgrid(
np.linspace(df["x0"].min(), df["x0"].max(), 200),
np.linspace(df["x1"].min(), df["x1"].max(), 100),
)
Z = qda.predict_proba(np.c_[xx.ravel(), yy.ravel()])
Z = Z[:, 1].reshape(xx.shape)
plt.pcolormesh(
xx, yy, Z, cmap=plt.get_cmap("bwr"), zorder=0, alpha=0.4
)
plt.contour(xx, yy, Z, [0.5], linewidths=2.0, colors="white")
g = sns.scatterplot(df["x0"], df["x1"], hue=df["y"])
/home/chansoo/projects/statsbook/.venv/lib/python3.8/site-packages/sklearn/base.py:439: UserWarning: X does not have valid feature names, but QuadraticDiscriminantAnalysis was fitted with feature names
warnings.warn(
/home/chansoo/projects/statsbook/.venv/lib/python3.8/site-packages/seaborn/_decorators.py:36: FutureWarning: Pass the following variables as keyword args: x, y. From version 0.12, the only valid positional argument will be `data`, and passing other arguments without an explicit keyword will result in an error or misinterpretation.
warnings.warn(
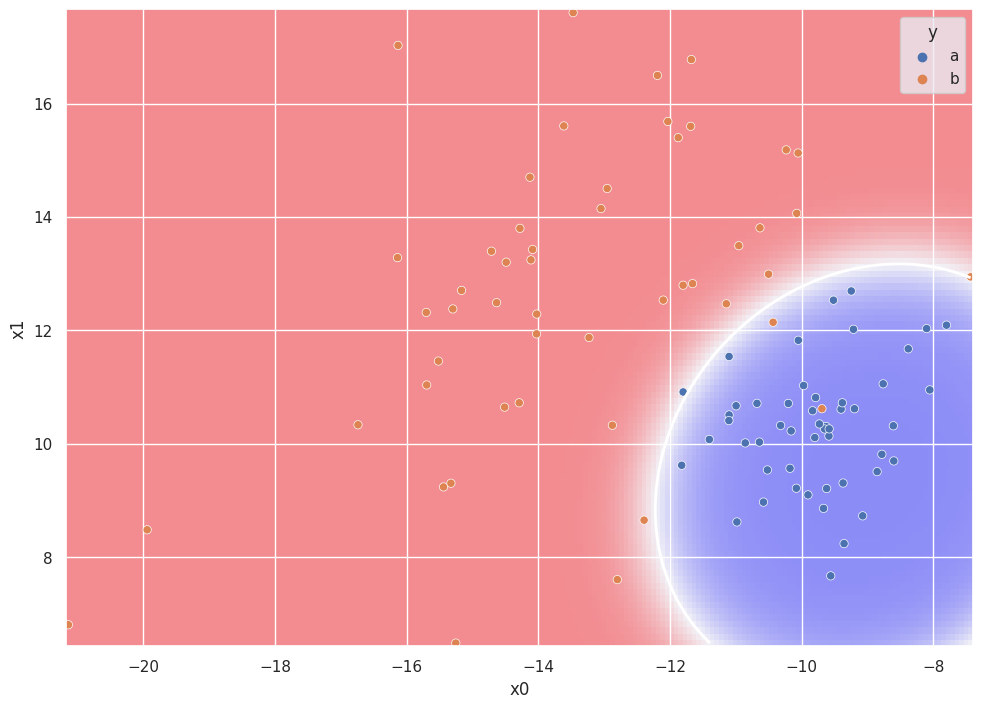
Model Evaluation#
# prediction
y_pred = qda.predict(df[["x0","x1"]])
#error rate
print(np.mean(df["y"]!=y_pred))
print(confusion_matrix(df["y"], y_pred))
# recall of the negative class is "specificity"
print(classification_report(df["y"], y_pred))
0.03
[[49 1]
[ 2 48]]
precision recall f1-score support
a 0.96 0.98 0.97 50
b 0.98 0.96 0.97 50
accuracy 0.97 100
macro avg 0.97 0.97 0.97 100
weighted avg 0.97 0.97 0.97 100